With the integration of artificial intelligence, winds of change have blown over the pharmaceutical industry, bringing in remarkable improvements in innovation and efficiency. However, things are not a cakewalk here. Merging AI with the pharma industry’s established legacy systems comes with its own set of challenges.
Although there are some major hurdles here, there are solutions too! And we’re here to discuss them. In this post, we’ll look at the complexities associated with AI integration in pharma legacy systems as well as the solutions to them.
Why is Integration of AI Difficult in Pharmaceutical Legacy Systems?
Integrating AI into legacy systems within the pharmaceutical industry presents significant challenges due to outdated technologies and complex data infrastructures. Legacy systems, typically built on rigid architectures, often fail to accommodate the flexible and dynamic requirements of AI-driven tools. These tools depend on seamless data integration and real-time processing. For instance, older systems may lack the ability to support real-time data analytics essential for predictive maintenance in manufacturing operations.
Additionally, legacy systems in the pharma industry manage critical data while complying with strict regulatory standards. This creates a dual challenge of ensuring that AI integration not only supports compliance but also drives innovation.
Common Legacy Systems and Challenges
In the pharma industry, legacy systems often include outdated batch process control systems and older ERP platforms. They lack the flexibility to integrate seamlessly with AI technologies. These systems are typically siloed, making it difficult to implement AI solutions requiring data from various functions to perform efficiently.
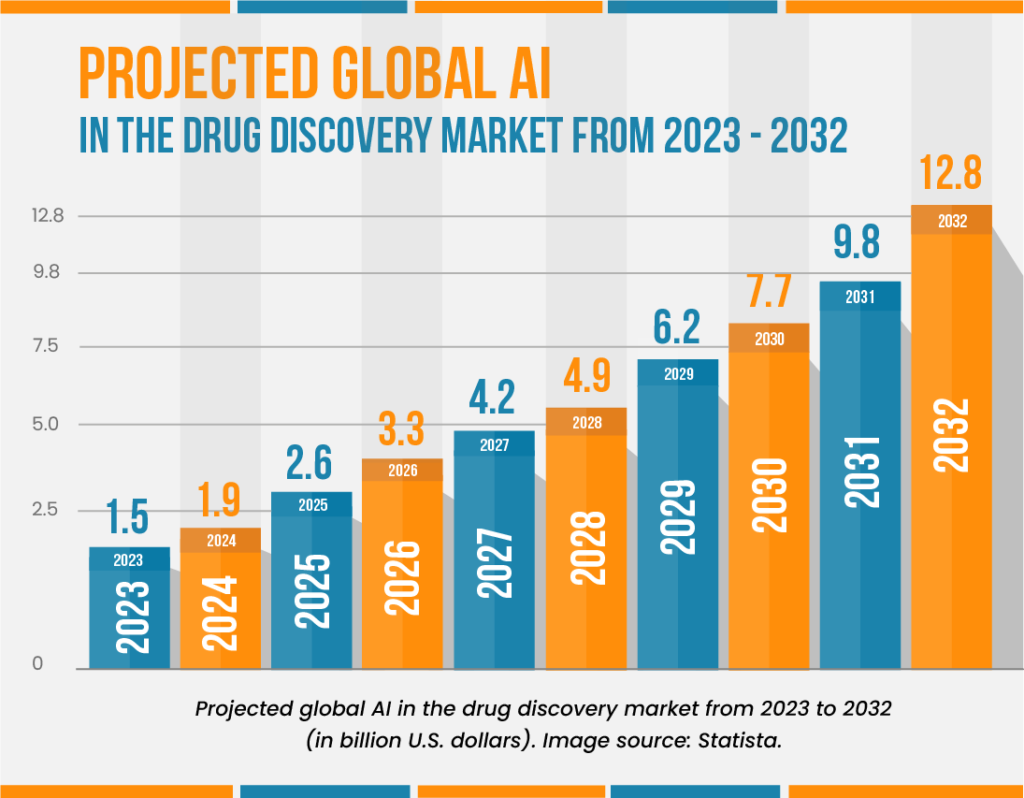
Advantages of Overcoming Integration Challenges: The Transformative Power of AI
Successfully integrating AI into these systems can bring transformative benefits to drug development and production. AI has the potential to optimize drug formulations and enhance predictive maintenance, minimizing downtime and boosting production efficiency. By enabling more advanced data analysis, AI integration supports better decision-making, leading to faster, more efficient drug development cycles.
Step-by-Step Guidance for AI Integration:
- Assessment and Planning: Analyze current systems to evaluate integration feasibility, identify capability gaps, and plan necessary upgrades or enhancements to support AI functionality.
- Collaborate with AI Technology Providers: Work with specialized AI solution providers in the pharmaceutical sector to develop tailored solutions that align with existing systems.
- Pilot Initiatives: Begin with pilot projects to test the integration in a controlled setting, addressing any challenges before implementing a full-scale rollout.
- Evaluate Data Architecture: Review the maturity of the current data architecture, including data warehouses, data lakes, and ETL processes. Ensure the architecture can support the volume, variety, and velocity of data required for AI-driven insights. Companies may need to modernize or expand their data infrastructure to enable seamless AI integration.
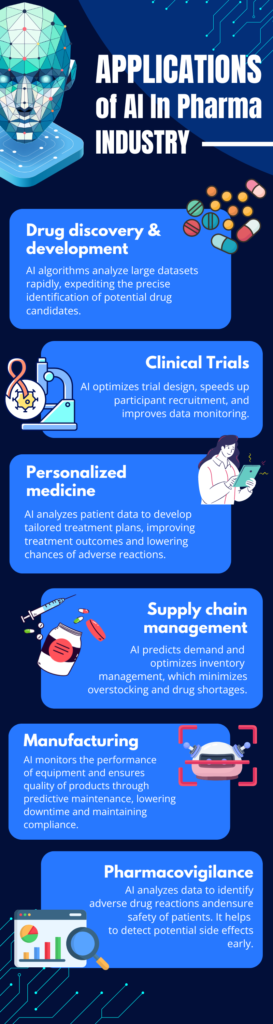
Examples and Case Studies
BenevolentAI and the COVID-19 pandemic
BenevolentAI, a biotech company based in the UK, leveraged AI to expedite drug discovery efforts for COVID-19. Their AI platform analyzed data from various sources, such as scientific research, clinical trials, and patient records, to uncover potential treatments. Using AI algorithms, BenevolentAI identified Baricitinib, a drug typically used for rheumatoid arthritis, as a promising option for treating COVID-19.
The selection of Baricitinib was based on its ability to inhibit a key protein linked to the inflammatory response caused by COVID-19. The drug was later authorized for emergency use in COVID-19 treatment.
Tempus and Cancer Treatment
Tempus, a technology company focused on precision medicine, utilizes AI to analyze genomic data and deliver personalized treatment recommendations for cancer patients.
By applying AI algorithms to genomic sequencing data, Tempus identifies mutations and patterns that guide treatment decisions. This enables specialists to customize treatment plans based on the unique genetic profile of each patient’s tumor.
Novartis:
Novartis is known for employing AI across drug discovery, clinical trials, and patient care. In collaboration with Microsoft, they use AI to process complex datasets and streamline clinical trials by identifying suitable patients and improving trial design.
Roche:
Roche is utilizing AI through its subsidiary, Flatiron Health, which focuses on oncology data. AI helps them process real-world data and extract valuable insights to speed up drug development, especially for cancer treatments.
GSK (GlaxoSmithKline):
GSK uses AI to speed up drug discovery and design better vaccines. Their partnership with AI firm Insilico Medicine is particularly focused on discovering new molecules and understanding biological pathways using AI.
What’s the Future of AI in Pharma?
The AI landscape is continually evolving, indicating that its influence and impact on the pharmaceutical industry could grow exponentially. Emerging trends and future advancements are poised to reshape AI’s role in this sector.
By leveraging AI techniques and advanced tools like quantum computing, researchers could simulate complex molecular interactions, accelerating the discovery of potential drug candidates.
AI platforms may increasingly incorporate real-time data from ongoing clinical trials, making drug development more dynamic and adaptive.
Advances in genomics and other omics could further enhance AI’s capabilities by analyzing comprehensive biological datasets to identify new biomarkers and therapeutic targets. This could drive progress in personalized medicine, enabling more precise treatments tailored to individual genetic profiles and disease mechanisms.
More sophisticated predictive analytics models could also enhance clinical trials by improving patient response forecasting, optimizing trial protocols, and identifying potential issues.
Finally, as AI becomes more integrated into core pharmaceutical processes, greater emphasis should be placed on ethical AI and explainability. Future developments must aim to make AI-driven decisions more transparent and comprehensible, ensuring responsible AI use and creating outputs that are easily interpretable by humans.
To Sum Up
AI technology is revolutionizing the pharmaceutical industry by improving drug discovery, development, and personalized medicine through increased efficiency and advanced data analysis. Its application can accelerate treatment identification and streamline clinical trials, ultimately enhancing patient outcomes.
However, challenges such as data privacy, biases, regulatory hurdles, and ethical concerns regarding decision-making must be addressed. As AI continues to evolve, the pharmaceutical sector is likely to see more advancements, with a critical focus on ethical AI practices and transparent, explainable decision-making.
AQB Solutions, with its extensive experience in AI and Data Science, can help you mitigate AI integration challenges in pharma, and reap the maximum benefits of AI use in the pharmaceutical domain.